Business Analytics
Accreditations
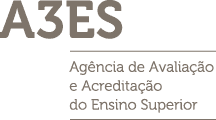
Programme Structure for 2024/2025
Curricular Courses | Credits | |
---|---|---|
1st Year | ||
Exploratory Data Analysis
6.0 ECTS
|
Parte Escolar > Mandatory Courses | 6.0 |
Unstructured Data Analytics
6.0 ECTS
|
Parte Escolar > Mandatory Courses | 6.0 |
Business Analytics Applications
6.0 ECTS
|
Parte Escolar > Mandatory Courses | 6.0 |
Strategy and Reporting
6.0 ECTS
|
Parte Escolar > Mandatory Courses | 6.0 |
Database Management
6.0 ECTS
|
Parte Escolar > Mandatory Courses | 6.0 |
Predictive Analytics
6.0 ECTS
|
Parte Escolar > Mandatory Courses | 6.0 |
Prescriptive Analytics
6.0 ECTS
|
Parte Escolar > Mandatory Courses | 6.0 |
Big Data Analytics
6.0 ECTS
|
Parte Escolar > Mandatory Courses | 6.0 |
Seminar in Business Analytics
6.0 ECTS
|
Parte Escolar > Mandatory Courses | 6.0 |
2nd Year | ||
Research Project Seminar in Business Analytics
6.0 ECTS
|
Parte Escolar > Mandatory Courses | 6.0 |
Master Dissertation in Business Analytics
42.0 ECTS
|
Final Work | 42.0 |
Master Project in Business Analytics
42.0 ECTS
|
Final Work | 42.0 |
Exploratory Data Analysis
At the end of the curricular period, the student must:
LG1. Apply the concepts inherent to each of the phases of the CRISP-DM methodology concepts with real data.
LG2. Understand and apply summarization techniques.
LG3. Understand and apply segmentation techniques.
LG4. Understand and apply association techniques.
P1. Exploratory data analysis
P1.1. Data classification
P1.2. Data quality: missing values and outliers
P2. Summarization techniques:
P2.1. Descriptive measures
P2.2. Graphs
P2.3. Principal Components Analysis
P3. Segmentation techniques
P4. Association techniques
P5. Applications of descriptive techniques with real data, using IBM SPSS Modeler and IBM SPSS Statistics; or others.
1) Periodic evaluation:
a) 2 individual Exercises realized in class with a weight of 15% each with a minimum grade of 8,5 points (out of 20)
b) A written individual final test with 70% of weight and a minimum grade of 8,5 points (out of 20)
The periodic evaluation requires attendance in at least 2/3 of the classes and approval will be obtained by students with a final minimum overall grade of 10, provided that they did not have a grade lower than 8.5 in any of the written individual tests.
2) Exam: Exam with a theoretical component (70%) + Exam with a practical component (30%), requiring a min grade of 8,5 in each exam, provided that the final classification is at least 10 points.
Title: Larose, D. T., Data Mining and Predictive Analytics, 2015, Wiley Series on Methods and Applications, 2nd Ed., ISBN: 978-1-118-11619-7
Camm, J., Cochran, J., Fry, M., Ohlmann, J., Anderson, D., Sweeney, D., & Williams, T., Essentials of Business Analytics, 2015, Cengage Learning, 2nd Ed., ISBN- 10 1305627733
Hair, J. H., Black, W. C., & Babin, B. J., Multivariate Data Analysis: A Global Perspective, 2010, Pearson Education, ISBN 0135153093, 9780135153093
Authors:
Reference:
Year:
Title: Laureano, R. & Botelho, M., IBM SPSS Statistics: o Meu Manual de Consulta Rápida, 2017, 4ª Edição, Edições Sílabo, ISBN 978-972-618-886-5
Raul Laureano, Testes de Hipóteses com o IBM SPSS Statistics: O Meu Manual, 2022, Edições Sílabo, 3ª ed., ISBN 978-989-561-052-5
Authors:
Reference:
Year:
Unstructured Data Analytics
At the end of this learning unit?s term, the student must be able to:
LG1. To identify and apply concepts and technologies associated with text mining and social network analysis in order to recommend action or to guide decision making rooted in unstructured data;
LG2. To apply text mining techniques to better understand and handle existing business problems;
LG3. To develop the personal capacity of studying, searching and communicating at the level of Text Mining.
PC1. Introduction to Text Mining
PC2. Tokenization, Dictionary Creation and Corpus Preparation
PC3. Clustering Methods for Text Mining
PC4. Practical cases on using clustering methods in text mining for business
PC5. Classification Methods for Text Mining
PC6. Practical cases on using classification methods in text mining for business
PC7. Sentiment Analysis
PC8. Practical cases on using sentiment analysis for business
1) Continuous evaluation:
a) Written test (50%)
(LG 1, 2, 3)
b) A group coursework (30%)
(LG 1, 2, 3)
c) Participation in class (10%)
Requires a minimum grade of 7.5 points in each element, attendance to classes of at least 2/3, and a minimum of 10 points in the final classification.
2) Exam (1st sitting): written test (100%), requiring minimum 10 points to get approval.
3) Exam (2nd sitting): written test (100%), requiring minimum 10 points to get approval.
Scale: 0-20 points.
Title: Struhl, S. (2015). Practical Text Analytics: Interpreting Text and Unstructured Data for Business Intelligence (Marketing Science Series). Kogan Page.
Artigos científicos indicados pelo docente.
Folhas de apoio às aulas elaboradas pelo docente.
Authors:
Reference:
Year:
Title: Weiss, Indurkhya, Zhang, Damerau (2005). Text Mining: Predictive Methods for Analyzing Unstructured Information. Springer
Srivastava, A.N. and Sahami, M. (2009). Text Mining: Classification, Clustering, and Applications. Chapman & Hall/CRC.
Feldman, R. and James Sanger, J. (2006). The Text Mining Handbookx\: Advanced Approaches in Analyzing Unstructured Data. Cambridge University Press.
Authors:
Reference:
Year:
Business Analytics Applications
LO1. To know CRISP-DM methodology
LO2. To understand the framework to implement business analytics projects
LO3. To know the main computer tools for business analytics
LO4. To know same business analytics applications
P.1. Business analytics in the organizational context
P1.1. Framework to implement
P1.2. CRISP-DM methodology
P1.3. Analytics maturity
P2. Business analytics applications
P2.1. Data problems
P2.2. Business intelligence problems
P2.3. Analytics problems
1) Periodic evaluation:
a) Individual work with oral and digital presentation (70%)
(LO 1, 2, 3, 4)
b) Group work with possible discussion (30%)
(LO 3, 4)
Requires a minimum grade of 7.5 points in each element, attendance to classes of at least 4/5, and a minimum of 10 points in the final classification
Scale: 0-20 points
Given the practical nature of the content of the course, no exam is scheduled.
Title: Camm, J., Cochran, J., Fry, M., Ohlmann, J., Business Analytics, 2021, Cengage,
Laursen, Gert H. N. & Thorlund, Jesper, Business Analytics for Managers: Taking Business Intelligence Beyond Reporting, 2017, Second Edition, Wiley,
Santos, M. & Ramos, I., Business Intelligence: Tecnologias da Informação na Gestão de Conhecimento, 2009, 2ª Edição, FCA.,
Schniederjans, M. J., Schniederjans, D. G., & Starkey, C. M., Business analytics principles, concepts, and application what, why, and how, 2014, Pearson,
Venkatesan, R, Farris, P. Wilcox R., Cutting Edge Marketing Analytics: Real World Cases and Datasets for Hands On Learning, 2014, Pearson/FT Press,
Authors:
Reference:
Year:
Title: Brennan, K., A Guide to the Business Analysis Body of Knowledge (BABOK Guide), 2015, V3, Int'l Institute of Business Analysis (IIBA),
Isson, J. P. & Harriott, J. (eds), Win with advanced business analytics: creating business value from your data, 2013, John Wiley & Sons,
Palmatier, Robert & Sridhar, Shrihari, Marketing Strategy: Based on First Principles and Data Analytics, 2021, 2nd Edition, Bloomsbury Academic,
Sharda, Ramesh, Dursun Delen & Efraim Turban, Business Intelligence and Analytics: Systems for Decision Support, 2015, 10th Edition, Pearson.,
Siegel, E., Predictive Analytics: The Power to Predict Who Will Click, Buy, Lie, or Die, 2016, Wiley,
Winston, Wayne L., Marketing Analytics Data-Driven Techniques with Microsoft Excel, 2014, Wiley,
Authors:
Reference:
Year:
Strategy and Reporting
LG1. To develop some of the concepts underlying the strategic management process and understand the ways different theoretical perspectives approach this process.
LG2. To understand and apply the concept of dynamic capabilities and its approach to strategy.
LG3. To introduce some of the concepts underlying the formulation and development of business and corporate strategies, including business environment evaluation and stakeholder analysis.
LG4. To understand the importance of implementation and control in the strategic management process and its relationship with reporting and business analytics.
LG5. To create control systems for performance evaluation and information reporting for business management.
LG6. To develop critical thinking.
LG7. To have autonomy to plan learning processes and advance knowledge in the area under study.
T1. Strategy as starting point for business analytics.
T2. The strategic management process.
T3. Internal analysis of the organization based on its resources and dynamic capabilities.
T4. External environment analysis.
T5. Business strategies, new business models and digital transformation.
T6. Corporate strategies.
T7. Strategy implementation and control, reporting and business analytics.
Option 1 (Continuous Assessment)
i. Individual Written Test 50%
ii. Group Assignment 40%
iii. Seminar Report 10%
To successfully complete the course, students must obtain a weighted average of 10 points or more, with at least 8 in each component of the continuous assessment.
Option 2 (Final Exam)
To pass: 10 or more points.
20-point scale.
Title: Sharda, R., Delen, D., & Turban, E. (2018) Business intelligence, analytics, and data science: A managerial perspective. Pearson.
Pearce, J. & Robison, R. (2013) Strategic Management, 13th Edition, MacGraw-hill Higher Education.
Grant, R. (2018) Contemporary Strategy Analysis (10th edition), Wiley.
Barney, J. & Hesterley S. (2019) Strategic Management and Competitive Advantage: Concepts and Cases, 6th Edition, Pearson.
Authors:
Reference:
Year:
Title: Gebauer, H. (2011) Exploring the contribution of management innovation to the evolution of dynamic capabilities. Industrial Marketing Management, 40(8), 1238-1250.
Eisenhardt, K. & Martin, J. (2000) Dynamic capabilities: What are they? Strategic Management Journal, 21(10/11), 1105-1121.
Breznik, L. & D. Hisrich, R. (2014) Dynamic capabilities vs. innovation capability: Are they related? Journal of Small Business and Enterprise Development, 21(3), 368-384.
Authors:
Reference:
Year:
Database Management
1. Data base management background in the data-science universe
2. Develop data modeling capabilities in the context of operational and analytical systems.
3. Ability to use with efficiency and effectiveness the languages and tools for information research in structured and semi-structured data
A. Data management background in the data-science universe
B. Relational Schemas Design
1. Relations and primary keys
2. Foreign keys and rules of integrity;
3. Critical analysis and construction of a relational model;
C. Language S.Q.L
1 Simple Interrogations;
2 Aggregation and Grouping Functions;
3 Chained Interrogations;
4. Creation of Views
D. Optimization
E. Dimensional Model
1. Dimensional model design
2. Dimensional vs relational model
3. ETL & data quality
F. Semi-structured data
1st sitting
1)Periodic evaluation:
i) group work with phased deliveries and discussion (50%);
ii) individual written test (50%).
Approval: Minimum final score of 10 values; minimum written test score 8 values.
2)Evaluation by exam: written test (100%), minimum score 10 values.
2nd sitting
Evaluation by exam: written test (100%), minimum score 10 values.
Scale: 0-20 values.
Title: Ramakrishnan , Raghu; Gehrke, Johannes. Database Management Systems. 3rd Edition. McGrawHill. 2003
Perreira, J. Tecnologia de Base de Dados" FCA Editora de Informática, 1998
Damas, L. SQL - Structured Query Language " FCA Editora de Informática, 2005
Kimball R, Ross M. The Data Warehouse Toolkit. 3rd ed. John Wiley & Sons; 2013.
Kimball R, Caserta J, ?The Data Warehouse ETL Toolkit?, Wiley, 2004
Authors:
Reference:
Year:
Title: Gorelik, Alex. The Enterprise Big Data Lake: Delivering the Promise of Big Data and Data Science. 1st Edition, O?Reilly, 2019
Authors:
Reference:
Year:
Predictive Analytics
LO1. Identify variables and metrics relevant to classification and regression and define the goals of the analysis
LO2. Classification techniques: decision trees, propositional rules and neural networks
LO3. Regression techniques: linear regression, decision trees and neural networks
LO4. Applications of classification and regression techniques on real data
P1. Exploratory data analysis and metrics for classification and regression
P2. Classification techniques:
P2.1. Decision trees and propositional rules
P2.2 Neural networks: the backpropagation algorithm
P2.3 Other algorithms for classification problems
P3.1. Linear regression
P3.2. Decision trees
P3.3 Neural networks: the backpropagation algorithm
P3.4. Other algorithms for regression problems
P4. Applications of classification and regression with real data, using IBM SPSS Modeler and IBM SPSS Statistics; or other
1) Periodic evaluation:
a) Written test (50%)
(LO 1, 2, 3)
b) A group project with digital presentation (50%) and possible discussion
(LO 4)
Requires a minimum grade of 7.5 points in each element, attendance to classes of at least 2/3, and a minimum of 10 points in the final classification.
2) Exam (1st sitting): project with discussion and digital presentation (50%) and written test (50%), requiring minimum 10 points in each assignment to get approval.
3) Exam (2nd sitting): project with discussion and digital presentation (50%) and written test (50%), requiring minimum 10 points each assignment to get approval.
Scale: 0-20 points.
Title: Larose, D. & Larose, C. (2015). Data Mining and Predictive Analytics (Wiley Series on Methods and Applications in Data Mining), 2nd edition, Wiley.
Lopez, C. (2022). Machine Learning. Supervised Learning with SPSS Modeler, Scientific Books.
Quinn, J. (2020). The Insider' Guide to Predictive Analytics, Smart Vision Europe.
Wendler, T. & Gröttrup, S. (2021). Data Mining with SPSS Modeler: Theory, Exercises and Solutions, 2nd edition, Springer
Witten, I., Frank, E. & Hall, M. (2011). Data Mining: Practical Machine Learning Tools and Techniques, 3rd edition, Morgan Kaufmann.
Authors:
Reference:
Year:
Title: Gama, J., Carvalho, A., Faceli, K., Lorena, A., & Oliveira, M. (2012). Extração de Conhecimento de Dados: Data Mining, Edições Sílabo.
Hair, J.F., Black, W.C., Babin, B.J. & Anderson, R.E. (2010). Multivariate Data Analysis, 7th edition, Prentice Hall.
Hastie, T., Tibshirani, R., Friedman, J., Hastie, T., Friedman, J., & Tibshirani, R. (2009). The Elements of Statistical Learning, Vol. 2, Springer.
Laureano, R. (2020). Testes de Hipóteses e Regressão: o Meu Manual de Consulta Rápida, Edições Sílabo.
McCormick, K., Abbott, D., Brown, M., Khabaza. T., & Mutchler, S. (2013). IBM SPSS Modeler Cookbook, Packt
Rocha, M., Cortez, P. & Neves, J. (2008). Análise Inteligente de Dados - Algoritmos e Implementação em Java, FCA.
Salcedo, J. & McCormick, K. (2017). IBM SPSS Modeler Essentials: Effective techniques for building powerful data mining and predictive analytics solutions, Packt.
Santos, M. & Ramos, I. (2009). Business Intelligence: Tecnologias da Informação na Gestão de Conhecimento, 2ª Edição, FCA.
Vasconcelos, J., & Barão, A. (2017). Ciência dos Dados nas Organizações: Aplicações em Python, FCA.
Authors:
Reference:
Year:
Prescriptive Analytics
At the end of this Curricular Unit, the student is expected to be able to:
LO1: Identify prescriptive decision support models appropriate for applications in management.
LO2: Identify prescriptive decision support techniques appropriate for applications in management.
LO3: Develop decision support models for applications in management.
LO4: Use general software to solve decision support models.
LO5: Interpret and produce recommendations based on the results of decision support models.
S1. Mathematical optimization models and techniques
1.1 Linear Optimization
1.2 Optimization with binary variables
1.3. Nonlinear Optimization
1.4 Applications of mathematical optimization in Management
1.5 Resolution using software
1.6 Make prescriptive recommendations
S2. Multiobjective optimization models and techniques
2.1 Modelos multiobjectivo
2.2 Applications of multiobjective optimization in Management
2.3 Resolution using software
2.4 Make prescriptive recommendations
S3. Heuristic optimization techniques
3.1 Introduction to heuristic optimization
3.2 Applications of heuristic optimization in Management
3.3 Resolution using software
3.4 Make prescriptive recommendations
S4. Other prescriptive models and techniques for Management
1. PERIODIC ASSESSMENT:
a) Written test (60%);
b) Group coursework with discussion (40%);
c) Attendance of at least 2/3 of the classes.
2. ASSESSMENT BY EXAM (1st and 2nd Season):
a) Written test (60%);
b) Individual project with discussion (40%);
Approval (in the Periodic or Exam evaluation):
i) Requires a minimum mark of 8.5 in each examination;
ii) An oral discussion may be required.
Scale: 0-20 points.
Title: * Evans, J. (2021). Business Analytics, 3rd Ed. Global Edition. Pearson.
* Ragsdale, C.T. (2017). Spreadsheet Modeling and Decision Analysis: A Practical Introduction to Business Analytics, 8th Ed. Cengage Learning.
* Murty, K. G. (2003). Optimization Models For Decision Making: Volume 1. Web-book. http://www-personal.umich.edu/~murty/books/opti_model/
Authors:
Reference:
Year:
Title: * Greasley, A. (2019) Simulating Business Processes for Descriptive, Predictive and Prescriptive Analytics, De Gruyter.
* Borshchev, A. (2015). The Big Book of Simulation Modeling. Multimethod Modeling with AnyLogic 6.
* Taha, H. A. (2016). Operations Research: An Introduction, 10th Ed. Pearson.
* Korte, B. and Vygen, J. (2012). Combinatorial Optimization: Theory and Algorithms, 5th edition. Springer.
* Pinedo, M.L. (2012). Scheduling: Theory, Algorithms, and Systems, 4th edition. Springer.
* Cook, J.W. (2014). In Pursuit of the Traveling Salesman: Mathematics at the Limits of Computation, 3rd Ed. Princeton University Press.
Authors:
Reference:
Year:
Big Data Analytics
LG1. Understand the basic concepts of Big Data and its implications in different fields of management
LG2. Apply analytical models with Big Data
LG3. Evaluate alternative analytical solutions with Big Data
P1. Big Data: Introduction, challenges, trends and applications
P2. Big Data characterization: The V's of Big Data
P3. Big data technologies
P4. Stream Analysis and analytical models for Big Data
P5. Business cases about Big Data problems and analytical solutions
1. Periodic evaluation: a) Group assignment with presentation (50%). (LG 2, 3); b) Individual test (50%). (LG 1, 2, 3).
Approval by periodic evaluation requires:
- students attendance of at least 75% of classes;
- minimum grade of 7,5 in the individual test and the group assignment;
- minimum final grade of 10.
2. Evaluation by exam: Individual test (50%) and Individual assignment with presentation (50%).
Approval by exam requires:
- minimum grade of 10 for each of the evaluation elements;
- minimum final classification of 10.
Title: Bahga, A. & Madisetti, V. (2016). Big Data Science & Analytics: A Hands-On Approach. VPT.
Li, K. C., Jiang, H., Yang, L. T., & Cuzzocrea, A. (2015). Big data: Algorithms, analytics, and applications. CRC Press.
Leskovec, J., Rajaraman, A. & Ullman, J.D. (2020). Mining of Massive Datasets. 3rd Edition, Cambridge University Press.
Melcher, K. & Silipo, R. (2020) Codeless Deep Learning with KNIME. Packt Publishing.
Authors:
Reference:
Year:
Title: Berthold, M.R., Borgelt, C., Höppner, F., Klawonn, F. & Silipo, R. (2020). Guide to Intelligent Data Science: How to Intelligently Make Use of Real Data. 2nd Edition, Springer International Publishing.
Marz, N. & Warren, J. (2015). Big Data: Principles and best practices of scalable realtime data systems. Manning Publications.
Ragsdale, C. (2015). Spreadsheet Modeling & Decision Analysis: A practical introduction to business analytics. 7th Edition, Cengage Learning.
Ryza, S., Laserson, U., Owen, S., & Wills, J. (2015). Advanced Analytics with Spark: Patterns for Learning from Data at Scale. O'Reilly Media.
Authors:
Reference:
Year:
Seminar in Business Analytics
LG1. To understand the key concepts and methodologies of a business case
LG2. To formulate an analytical business case
LG3. To deliver and communicate an analytical business case
P1. Business case concept and elements
P1.1. Fundaments of project management
P1.2. The main frameworks
P2. Types of analytical business cases
P3. The role of consulting
P3.1. Analytical business case design
P3.2. Analytical business case delivery
P4. Analytical business cases examples
Individual written work and its presentation and discussion (50%)
(LG 1, 2, 3)
Team work and its presentation and discussion (50%)
(LG 1, 2, 3)
Requires a minimum grade of 10 points in both works, and attendance to classes of at least 2/3.
Scale: 0-20 points.
There is only no final exam in this curricular unit.
Title: Wysocki, Robert. Effective Project Management: Traditional, Agile, Extreme, Hybrid. 8th edition. Wiley.
Schmidt, Marty. Business Case Guide. Everything you need to know about business case analysis. Solution Matrix. 3rd edition. 2020.
Power, Daniel and Heavin, Ciara. Decision Support, Analytics, and Business Intelligence. 3rd Edition. Business Expert Press. 2017.
Finlay, Steven. Artificial Intelligence and Machine Learning for Business: A No-Nonsense Guide to Data Driven Technologies. 3rd Edition. Relativistic, 2018.
Blokdyk, Gerardus. Design sprint: Standards Requirements. CreateSpace Publishing. 2018.
Authors:
Reference:
Year:
Research Project Seminar in Business Analytics
LG1. To know how to differentiate: a dissertation and a business analytics project
LG2. To understand the philosophy that underpins the choices and decisions to be made in taking a research position
LG3. To know how to select a research problem and review the literature
LG4. To know how to write a business analytics thesis project
LG5. To know how to communicate a research problem to managers
P.1 The stages of research
P1.1.Types of thesis
P1.2. Identifying the research problems
P1.3. Planning the research stages
P2. Doing a literature review and identifying the main literature sources
P3. Types and stages of empirical work in business analytics
P3.1. Identifying and using the business analytics tools to solve the research problem
P4. Research topics in business analytics
P5. Development and formal presentation of the research project
P5.1. Tutorial and individual orientation, supporting each student in the development of his project
Periodic assessment:
- Thesis project with oral and digital presentation (70%)
- Critical review of a business analytics research (10%)
- Protocol for literature review in the format of a scientific article with, possible, oral presentation (20%)
Requires a min. grade of 7.5 pts. in each element, attendance to classes of at least 2/3, and a min. of 10 pts. in the final classification.
Scale: 0-20 points.
This course does not have a final exam.
Title: Chapman, P., Clinton, J., Kerber, R., Khabaza, T., Reinartz, T., Shearer, C. & Wirth, R., CRISP-DM 1.0 - Step-by-step Data Mining Guide, 2000, The CRISP-DM consortium,
IBS, Regras de Elaboração de Dissertação ou Trabalho de Projeto de Mestrado, 2020, Iscte-Business School, https://www.iscte-iul.pt/assets/files/2020/10/28/1603893748579_Normas_elaborac_a_o_teses_de_mestrado_PT_IBS_Versa_o_Set_2020.pdf
Kitchenham, B., Procedures for Performing Systematic Reviews, Joint Technical Report TR/SE-0401, 2004, Keele University, https://www.researchgate.net/publication/228756057_Procedures_for_Performing_Systematic_Reviews
Peffers, K., Tuunanen, T., Gengler, C., Rossi, M., Hui, W., Virtanen, V., & Bragge, J., The Design Science Research Process: A Model for Producing and Presenting Information Systems Research,, 2006, First International Conference on Design Science Research in Information Systems and Technology (DESRIST 2006),
Saunders, M., Lewis, P., & Thornhill, A., Research Methods for Business Students, 2019, 8th Edition, Pearson,
vom Brocke, J., Hevner, A., & Maedche, A. (Eds.), Design Science Research. Cases, 2020, Springer,
Ildefonso, M., Laureano, R. & Vasarhelyi, M., Modelos preditivos de insolvências: uma revisão sistemática da literatura, 2023, 18th Iberian Conference on Information Systems and Technologies (CISTI), https://doi.org/10.23919/CISTI58278.2023.10211516
Authors:
Reference:
Year:
Title: Bhattacherjee, A., Social Science Research: Principles, Methods, and Practices, 2012, 2nd edition, University of South Florida, Scholar Commons, https://open.umn.edu/opentextbooks/formats/103
Flick, Uwe, An Introduction to Qualitative Research, 2023, 7th Edition, Sage Publications,
Gastel, B., & Day, R., How to Write and Publish a Scientific Paper, 2016, 8th Edition, Greenwood,
Oliveira, L. A., Dissertação e Tese em Ciência e Tecnologia Segundo Bolonha, 2011, Lidel,
Roger, B. & Sekaran, U., Research Methods for Business,, 2020, 8ª edição,, Wiley,
Authors:
Reference:
Year:
Master Dissertation in Business Analytics
LG1. Writing a dissertation
LG2. Writing a synthesis of the thesis
LG3. Public oral presentation of the synthesis of the thesis
P1. Writing the introduction and abstract (resumo);
P2. Definition of a research problem;
P3. Definition of research goals;
P4. Literature review;
P5. Defining hypothesis or the analytical objectives and monitoring metrics;
P6. Data collection methods and/or data understanding and preparation;
P7. Data analysis methods (modelling) and evaluation;
P8. Writing conclusions and defining new research paths;
P9. Recommendations and Implications in academic and practical terms
- Written presentation of the dissertation (80%)
- Oral presentation with the synthesis of the dissertation followed by a public defense with a jury (20%)
Title: Camm, J., Cochran, J., Fry, M., Ohlmann, J., Anderson, D., Sweeney, D., & Williams, T. (2015). Essentials of Business Analytics, Cengage Learning.
Uma Sekaran e Bougie Roger (2010) Research Methods for Business, 5ª edição, John Wiley and Sons
Oliveira, Luís Adriano (2011). Dissertação e Tese em Ciência e Tecnologia Segundo Bolonha. Lisboa: LIDEL
Laursen, Gert & Thorlund, Jesper (2010) Business Analytics for Managers: Taking Business Intelligence Beyond Reporting, Wiley.
Fisher, C. (2007). Researching and writing a dissertation: A guidebook for business students. Essex: Prentice Hall
Definida pelo orientador / Defined by supervisor
Authors:
Reference:
Year:
Title: Provost, F., & Fawcett, T. (2013). Data Science for Business Fundamental principles of data mining and data-analytic thinking. Sebastopol, CA: O?Reilly.
Pidd, M. (2003). Tools for thinking: Modelling in Mangement Science. West Sussex: Wiley.
Brennan, K. (2009). A Guide to the Business Analysis Body of Knowledge (BABOK Guide). IIBA.
Øvretveit, J. (2008). Writing a scientific publication for a management journal. Journal of Health Organization and Management, 22, 2, 189-206.
Authors:
Reference:
Year:
Master Project in Business Analytics
LG1. Writing a master project
LG2. Writing a synthesis of the master project
LG3. Preparing a public oral presentation of the synthesis of the master project
P1. Writing the introduction and abstract (resumo);
P2. Definition of the business problem and diagnosis of organizational environment;
P3. Definition of the project goals;
P4. Applied literature review;
P5. Defining the analytical objectives and monitoring metrics;
P6. Data understanding and preparation;
P7. Data analysis methods (modelling) and evaluation;
P8. Writing conclusions and defining new projects paths;
P9. Evaluation of impacts and possibilities of control of results.
- Written presentation of the thesis (80%)
- Oral presentation with the synthesis of the project followed by a public defense with a jury (20%)
Title: Camm, J., Cochran, J., Fry, M., Ohlmann, J., Anderson, D., Sweeney, D., & Williams, T. (2015). Essentials of Business Analytics, Cengage Learning.
Uma Sekaran e Bougie Roger (2010) Research Methods for Business, 5ª edição, John Wiley and Sons
Oliveira, Luís Adriano (2011). Dissertação e Tese em Ciência e Tecnologia Segundo Bolonha. Lisboa: LIDEL
Laursen, Gert & Thorlund, Jesper (2010) Business Analytics for Managers: Taking Business Intelligence Beyond Reporting, Wiley.
Fisher, C. (2007). Researching and writing a dissertation: A guidebook for business students. Essex: Prentice Hall
Bell, Judith (2005). Doing Your Research Project: a guide for first-time researchers in education and social science. 4th ed. Buckingham: Open University Press.
Definida pelo orientador / Defined by supervisor
Authors:
Reference:
Year:
Title: Provost, F., & Fawcett, T. (2013). Data Science for Business Fundamental principles of data mining and data-analytic thinking. Sebastopol, CA: O?Reilly.
Pidd, M. (2003). Tools for thinking: Modelling in Mangement Science. West Sussex: Wiley.
Brennan, K. (2009). A Guide to the Business Analysis Body of Knowledge (BABOK Guide). IIBA.
Øvretveit, J. (2008). Writing a scientific publication for a management journal. Journal of Health Organization and Management, 22, 2, 189-206.
Authors:
Reference:
Year:
Recommended optative
1st Year Data Analysis and Data Communication with Excel (03281)
2st Year Business Analytics Overview (03282)
Objectives
The degree aims to endow students with the ability to know necessary analytical instruments and know-how to recommend and use them in academic and professional fields. It allows students to develop business solutions based on analytics. In particular, allows to acquire the skills needed to foster a smooth transition into the job market, namely:
The ability to communicate orally and to write analyses and conclusions, as well as the knowledge and the reasoning underlying them, both to experts and non-experts
The ability to show critical thinking skills, integrate knowledge, handle complex issues, develop solutions and make judgments in situations of limited or incomplete information
The ability to show knowledge of relevant theories and models in the field of BA, including its concepts, theories, methods, and techniques
The ability to solve problems in the field of business, apply the acquired knowledge and skills to identify and solve problems, in new or unfamiliar situations
The learning outcomes to be attained by students upon attendance of the theoretical-practical classes and the accomplishment of group works are, among others:
To produce a well-structured document and clearly identify the relevant key messages within a written document on a (business) analytics problem
To recognize the significance of data in management
To select and interpret data and references from academic and non-academic sources
To effectively analyze problems, outlining well-grounded conclusions or solutions
To reveal knowledge of the existing market analytical methodologies and tools
To understand the framework for a Business Analytics project and assess the success of projects
To exhibit proficiency in Business Analytics research and/or project development
To be acquainted with computer tools, statistical and analytics packages, either open-source or commercial, suitable for diverse business problems
Accreditations
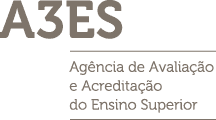